Text-to-SQL with LangChain (1) - Chain
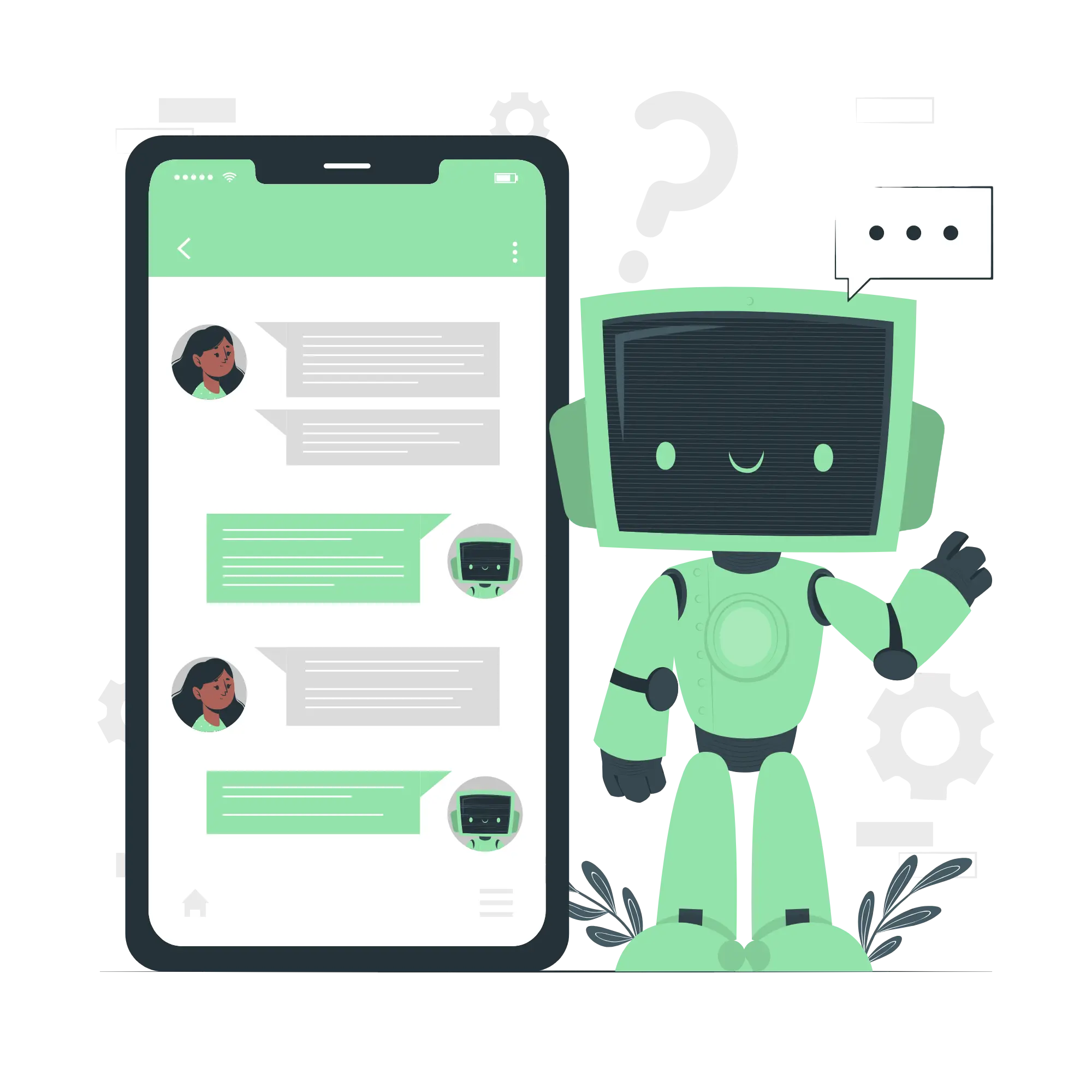
In this post, we’ll dive into how to set up Text-to-SQL with LangChain, guided by the Q&A over SQL+CSV Quickstart.
-
We’ll be using LangChain version
0.2.0
.$ pip list|grep langchain langchain 0.2.0 langchain-community 0.2.0 langchain-core 0.2.0 langchain-openai 0.1.7 langchain-text-splitters 0.2.0
1. Setup
1.1. Install Libraries
First, install the necessary libraries.
pip install --upgrade --quiet langchain langchain-community langchain-openai
1.2. Set Environment Variable OPENAI_API_KEY
Next, set the environment variable OPENAI_API_KEY
. Create a .openai
file in your working directory containing your OpenAI API key, and run the following code.
import os
# Set API key as an environment variable
with open('.openai') as f:
os.environ['OPENAI_API_KEY'] = f.read().strip()
1.3. Prepare a Test Database
For testing, we’ll use the Chinook database, a sample database that simulates a digital media store. It includes tables such as:
- Artists: Artist information
- Albums: Album information
- Tracks: Track (song) information
- Customers: Customer information
- Invoices: Invoice information
- Employees: Employee information
To create the Chinook database, download this file and place it in your working directory. Then, run the following code.
import sqlite3
# Database file name
db_file = 'Chinook.db'
sql_file = 'Chinook_Sqlite.sql'
# Open database connection
with sqlite3.connect(db_file) as conn:
cursor = conn.cursor()
# Read SQL file
with open(sql_file, 'r', encoding='utf-8') as file:
sql_script = file.read()
# Execute SQL script
cursor.executescript(sql_script)
# Commit and save database
conn.commit()
This will create a SQLite database named Chinook.db
in your working directory.
1.4. Test Connection
Let’s test the connection to the created SQLite database.
from langchain_community.utilities import SQLDatabase
# Specify the database to connect to
db = SQLDatabase.from_uri("sqlite:///Chinook.db")
print(db.dialect) # Database type
print(db.get_usable_table_names()) # List of usable table names
# Execute an SQL statement
db.run("SELECT * FROM Artist LIMIT 10;")
-
Expected output:
sqlite ['Album', 'Artist', 'Customer', 'Employee', 'Genre', 'Invoice', 'InvoiceLine', 'MediaType', 'Playlist', 'PlaylistTrack', 'Track']
"[(1, 'AC/DC'), (2, 'Accept'), (3, 'Aerosmith'), (4, 'Alanis Morissette'), (5, 'Alice In Chains'), (6, 'Antônio Carlos Jobim'), (7, 'Apocalyptica'), (8, 'Audioslave'), (9, 'BackBeat'), (10, 'Billy Cobham')]"
2. Chain
2.1. create_sql_query_chain
Using create_sql_query_chain
, we can create a chain that converts natural language queries into SQL statements.
from langchain.chains import create_sql_query_chain
from langchain_openai import ChatOpenAI
llm = ChatOpenAI(model="gpt-3.5-turbo", temperature=0)
chain = create_sql_query_chain(llm, db)
Invoke the created chain as follows:
response = chain.invoke({"question": "How many employees are there?"})
print(response)
-
Expected output:
SELECT COUNT("EmployeeId") AS "TotalEmployees" FROM "Employee"
Execute this SQL statement in the database to get the result.
db.run(response)
-
Expected output:
'[(8,)]'
2.2. Automatically Execute Generated SQL Statements
By chaining QuerySQLDataBaseTool
, we can automatically execute the generated query and get the result.
from langchain_community.tools.sql_database.tool import QuerySQLDataBaseTool
execute_query = QuerySQLDataBaseTool(db=db)
write_query = create_sql_query_chain(llm, db)
chain = write_query | execute_query
chain.invoke({"question": "How many employees are there?"})
-
Expected output:
'[(8,)]'
{"question": "Delete all data from the Album table."}
, the query to delete all data from the Album table will be executed automatically. Ensure proper permission management when using this in production.2.3. Answer in Natural Language
The chain we created above directly returns the SQL query result. To output the answer in natural language based on the query result, create a chain like this:
from operator import itemgetter
from langchain_core.output_parsers import StrOutputParser
from langchain_core.prompts import PromptTemplate
from langchain_core.runnables import RunnablePassthrough
answer_prompt = PromptTemplate.from_template(
"""Based on the user's question, the corresponding SQL query, and the SQL result, answer the user's question.
Question: {question}
SQLQuery: {query}
SQLResult: {result}
Answer: """
)
# Chain to generate answers using the above prompt
answer = answer_prompt | llm | StrOutputParser()
# Chain to pass the SQL query result to the answer chain
chain = (
RunnablePassthrough.assign(
query=write_query
).assign(
result=itemgetter("query") | execute_query
)
| answer
)
chain.invoke({"question": "How many employees are there?"})
-
Expected output:
'There are a total of 8 employees.'
2.4. Reviewing create_sql_query_chain
Code
Let’s review the code for the create_sql_query_chain
function to understand its workings (link).
def create_sql_query_chain(
llm: BaseLanguageModel,
db: SQLDatabase,
prompt: Optional[BasePromptTemplate] = None,
k: int = 5,
) -> Runnable[Union[SQLInput, SQLInputWithTables, Dict[str, Any]], str]:
"""Create a chain that generates SQL queries.
*Security Note*: This chain generates SQL queries for the given database.
The SQLDatabase class provides a get_table_info method that can be used
to get column information as well as sample data from the table.
To mitigate risk of leaking sensitive data, limit permissions
to read and scope to the tables that are needed.
Optionally, use the SQLInputWithTables input type to specify which tables
are allowed to be accessed.
Control access to who can submit requests to this chain.
See https://python.langchain.com/docs/security for more information.
Args:
llm: The language model to use.
db: The SQLDatabase to generate the query for.
prompt: The prompt to use. If none is provided, will choose one
based on dialect. Defaults to None. See Prompt section below for more.
k: The number of results per select statement to return. Defaults to 5.
Returns:
A chain that takes in a question and generates a SQL query that answers
that question.
Example:
.. code-block:: python
# pip install -U langchain langchain-community langchain-openai
from langchain_openai import ChatOpenAI
from langchain.chains import create_sql_query_chain
from langchain_community.utilities import SQLDatabase
db = SQLDatabase.from_uri("sqlite:///Chinook.db")
llm = ChatOpenAI(model="gpt-3.5-turbo", temperature=0)
chain = create_sql_query_chain(llm, db)
response = chain.invoke({"question": "How many employees are there"})
Prompt:
If no prompt is provided, a default prompt is selected based on the SQLDatabase dialect. If one is provided, it must support input variables:
* input: The user question plus suffix "\nSQLQuery: " is passed here.
* top_k: The number of results per select statement (the `k` argument to
this function) is passed in here.
* table_info: Table definitions and sample rows are passed in here. If the
user specifies "table_names_to_use" when invoking chain, only those
will be included. Otherwise, all tables are included.
* dialect (optional): If dialect input variable is in prompt, the db
dialect will be passed in here.
Here's an example prompt:
.. code-block:: python
from langchain_core.prompts import PromptTemplate
template = '''Given an input question, first create a syntactically correct {dialect} query to run, then look at the results of the query and return the answer.
Use the following format:
Question: "Question here"
SQLQuery: "SQL Query to run"
SQLResult: "Result of the SQLQuery"
Answer: "Final answer here"
Only use the following tables:
{table_info}.
Question: {input}'''
prompt = PromptTemplate.from_template(template)
""" # noqa: E501
if prompt is not None:
prompt_to_use = prompt
elif db.dialect in SQL_PROMPTS:
prompt_to_use = SQL_PROMPTS[db.dialect]
else:
prompt_to_use = PROMPT
if {"input", "top_k", "table_info"}.difference(prompt_to_use.input_variables):
raise ValueError(
f"Prompt must have input variables: 'input', 'top_k', "
f"'table_info'. Received prompt with input variables: "
f"{prompt_to_use.input_variables}. Full prompt:\n\n{prompt_to_use}"
)
if "dialect" in prompt_to_use.input_variables:
prompt_to_use = prompt_to_use.partial(dialect=db.dialect)
inputs = {
"input": lambda x: x["question"] + "\nSQLQuery: ",
"table_info": lambda x: db.get_table_info(
table_names=x.get("table_names_to_use")
),
}
return (
RunnablePassthrough.assign(**inputs) # type: ignore
| (
lambda x: {
k: v
for k, v in x.items()
if k not in ("question", "table_names_to_use")
}
)
| prompt_to_use.partial(top_k=str(k))
| llm.bind(stop=["\nSQLResult:"])
| StrOutputParser()
| _strip
)
-
If no
prompt
option is specified,SQL_PROMPTS[db.dialect]
is used. In this case,db.dialect
issqlite
, so the following prompt template is used.SQLITE_PROMPT = PromptTemplate( input_variables=["input", "table_info", "top_k"], template=_sqlite_prompt + PROMPT_SUFFIX, ) _sqlite_prompt = """You are a SQLite expert. Given an input question, first create a syntactically correct SQLite query to run, then look at the results of the query and return the answer to the input question. Unless the user specifies in the question a specific number of examples to obtain, query for at most {top_k} results using the LIMIT clause as per SQLite. You can order the results to return the most informative data in the database. Never query for all columns from a table. You must query only the columns that are needed to answer the question. Wrap each column name in double quotes (") to denote them as delimited identifiers. Pay attention to use only the column names you can see in the tables below. Be careful to not query for columns that do not exist. Also, pay attention to which column is in which table. Pay attention to use date('now') function to get the current date, if the question involves "today". Use the following format: Question: Question here SQLQuery: SQL Query to run SQLResult: Result of the SQLQuery Answer: Final answer here """ PROMPT_SUFFIX = """Only use the following tables: {table_info} Question: {input}"""
-
template
You are a SQLite expert. Given an input question, first create a syntactically correct SQLite query to run, then look at the results of the query and return the answer to the input question. Unless the user specifies in the question a specific number of examples to obtain, query for at most {top_k} results using the LIMIT clause as per SQLite. You can order the results to return the most informative data in the database. Never query for all columns from a table. You must query only the columns that are needed to answer the question. Wrap each column name in double quotes (") to denote them as delimited identifiers. Pay attention to use only the column names you can see in the tables below. Be careful to not query for columns that do not exist. Also, pay attention to which column is in which table. Pay attention to use date('now') function to get the current date, if the question involves "today". Use the following format: Question: Question here SQLQuery: SQL Query to run SQLResult: Result of the SQLQuery Answer: Final answer here Only use the following tables: {table_info} Question: {input}
-
-
The
llm
stops processing once it encounters\nSQLResult:
. Theinput
value contains “the value of thequestion
key +\nSQLQuery:
”, so only the corresponding SQL statement is expected to be output. -
db.get_table_info
outputs the table definitions and 3 rows of sample data.CREATE TABLE "Album" ( "AlbumId" INTEGER NOT NULL, "Title" NVARCHAR(160) NOT NULL, "ArtistId" INTEGER NOT NULL, PRIMARY KEY ("AlbumId"), FOREIGN KEY("ArtistId") REFERENCES "Artist" ("ArtistId") ) /* 3 rows from Album table: AlbumId Title ArtistId 1 For Those About To Rock We Salute You 1 2 Balls to the Wall 2 3 Restless and Wild 2 */
-
If the query doesn’t specify the number of rows, the default is 5. You can change this number with the
k
option. -
The generated chain allows you to specify the
table_names_to_use
key in the input dictionary to limit the tables used, saving token count.
table_names_to_use
key, the LLM might infer table names
from the query and access tables outside the specified list.
Do not use the table_names_to_use
key as a substitute for proper permission management.2.5. Summary
Here’s a summary of the code to query a database in natural language and get the answer in natural language.
import os
from operator import itemgetter
from langchain.chains import create_sql_query_chain
from langchain_community.tools.sql_database.tool import QuerySQLDataBaseTool
from langchain_community.utilities import SQLDatabase
from langchain_core.output_parsers import StrOutputParser
from langchain_core.prompts import PromptTemplate
from langchain_core.runnables import RunnablePassthrough
from langchain_openai import ChatOpenAI
# Set API key as an environment variable
with open(".openai") as f:
os.environ["OPENAI_API_KEY"] = f.read().strip()
# Specify the database to connect to
db = SQLDatabase.from_uri("sqlite:///Chinook.db")
# LLM settings
llm = ChatOpenAI(model="gpt-3.5-turbo", temperature=0)
# Tool to execute SQL statements
execute_query = QuerySQLDataBaseTool(db=db)
# Chain to generate SQL statements
write_query = create_sql_query_chain(llm, db)
# Prompt template to generate answers in natural language
answer_prompt = PromptTemplate.from_template(
"""Based on the user's question, the corresponding SQL query, and the SQL result, answer the user's question.
Question: {question}
SQLQuery: {query}
SQLResult: {result}
Answer: """
)
# Chain to generate answers using the above prompt
answer = answer_prompt | llm | StrOutputParser()
# Chain to pass the SQL query result to the answer chain
chain = (
RunnablePassthrough.assign(
query=write_query
).assign(
result=itemgetter("query") | execute_query
)
| answer
)
# Execute the query
chain.invoke({"question": "How many employees are there?"})