Reading LangChain's Summarization Code (3) - Refine
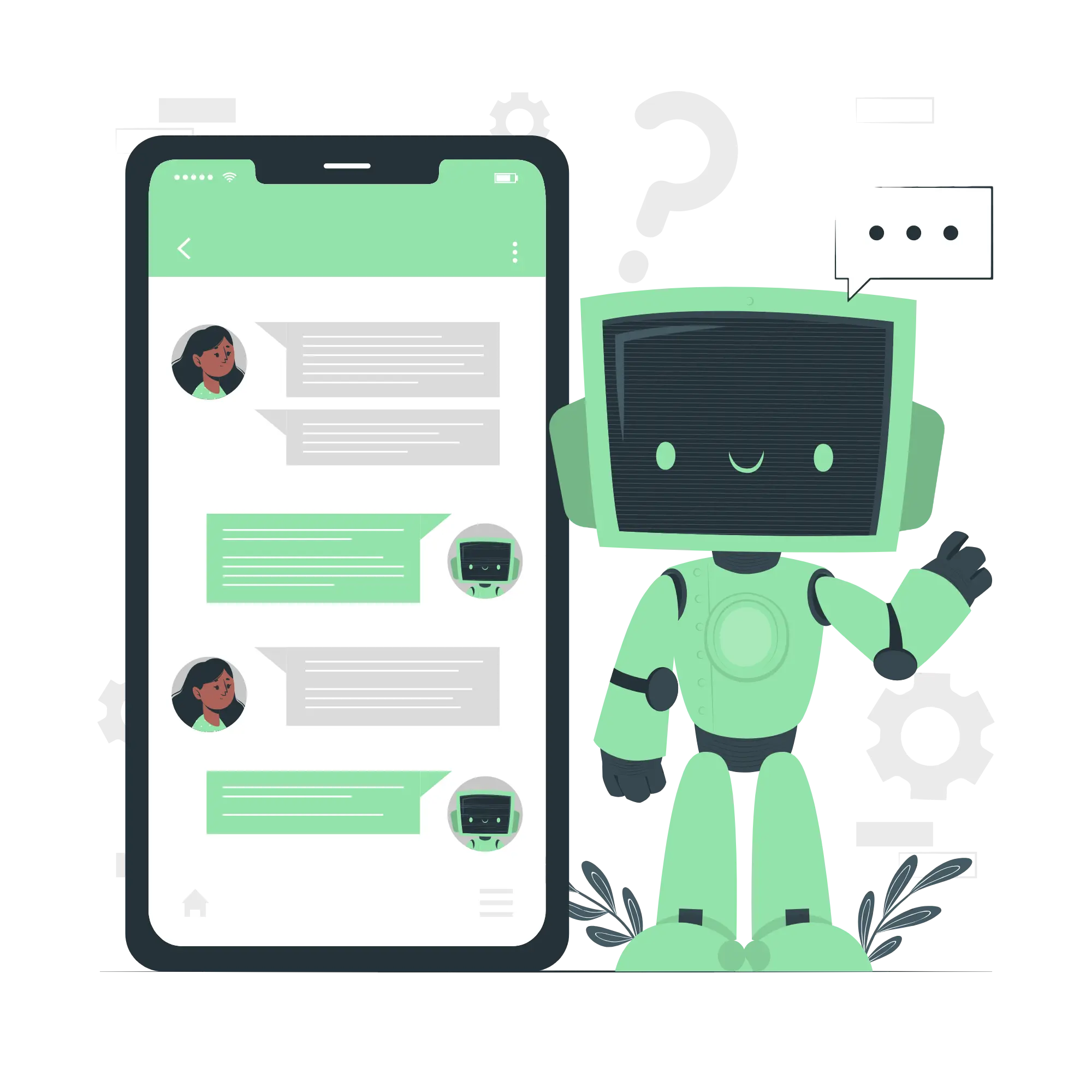
In this series, we explore the mechanism behind the text summarization chain introduced in LangChain’s Summarization documentation.
In this post, we focus on the Refine summarization method (chain_type="refine"
).
-
This article uses LangChain version
0.1.17
.$ pip list|grep langchain langchain 0.1.17 langchain-community 0.0.37 langchain-core 0.1.52 langchain-openai 0.1.6 langchain-text-splitters 0.0.1 langchainhub 0.1.15
Option 3: Refine
Let’s take a look at the code for the chain_type="refine"
scenario. The summarization chain in this case is generated by the following code:
_load_refine_chain(llm, verbose=verbose, **kwargs)
_load_refine_chain
The _load_refine_chain
function is defined as follows (link):
def _load_refine_chain(
llm: BaseLanguageModel,
question_prompt: BasePromptTemplate = refine_prompts.PROMPT,
refine_prompt: BasePromptTemplate = refine_prompts.REFINE_PROMPT,
document_variable_name: str = "text",
initial_response_name: str = "existing_answer",
refine_llm: Optional[BaseLanguageModel] = None,
verbose: Optional[bool] = None,
**kwargs: Any,
) -> RefineDocumentsChain:
initial_chain = LLMChain(llm=llm, prompt=question_prompt, verbose=verbose) # type: ignore[arg-type]
_refine_llm = refine_llm or llm
refine_chain = LLMChain(llm=_refine_llm, prompt=refine_prompt, verbose=verbose) # type: ignore[arg-type]
return RefineDocumentsChain(
initial_llm_chain=initial_chain,
refine_llm_chain=refine_chain,
document_variable_name=document_variable_name,
initial_response_name=initial_response_name,
verbose=verbose, # type: ignore[arg-type]
**kwargs,
)
-
It uses
LLMChain
to create aninitial_chain
that generates answers using the specified LLM (llm
) and prompt template (question_prompt
).-
By default,
question_prompt
is set torefine_prompts.PROMPT
, defined as follows (link):prompt_template = """Write a concise summary of the following: "{text}" CONCISE SUMMARY:""" PROMPT = PromptTemplate.from_template(prompt_template)
-
-
Similarly, it creates a
refine_chain
that generates answers using the specified LLM (refine_llm
) and prompt template (refine_prompt
).-
If
refine_llm
is not specified,llm
is used. -
By default,
refine_prompt
is set torefine_prompts.REFINE_PROMPT
, defined as follows (link):REFINE_PROMPT_TMPL = """\ Your job is to produce a final summary. We have provided an existing summary up to a certain point: {existing_answer} We have the opportunity to refine the existing summary (only if needed) with some more context below. ------------ {text} ------------ Given the new context, refine the original summary. If the context isn't useful, return the original summary.\ """ # noqa: E501 REFINE_PROMPT = PromptTemplate.from_template(REFINE_PROMPT_TMPL)
-
-
It then returns a
RefineDocumentsChain
usinginitial_chain
andrefine_chain
.
RefineDocumentsChain
Let’s take a look at the RefineDocumentsChain
code (link).
class RefineDocumentsChain(BaseCombineDocumentsChain):
"""Combine documents by doing a first pass and then refining on more documents.
This algorithm first calls `initial_llm_chain` on the first document, passing
that first document in with the variable name `document_variable_name`, and
produces a new variable with the variable name `initial_response_name`.
Then, it loops over every remaining document. This is called the "refine" step.
It calls `refine_llm_chain`,
passing in that document with the variable name `document_variable_name`
as well as the previous response with the variable name `initial_response_name`.
Example:
.. code-block:: python
from langchain.chains import RefineDocumentsChain, LLMChain
from langchain_core.prompts import PromptTemplate
from langchain_community.llms import OpenAI
# This controls how each document will be formatted. Specifically,
# it will be passed to `format_document` - see that function for more
# details.
document_prompt = PromptTemplate(
input_variables=["page_content"],
template="{page_content}"
)
document_variable_name = "context"
llm = OpenAI()
# The prompt here should take as an input variable the
# `document_variable_name`
prompt = PromptTemplate.from_template(
"Summarize this content: {context}"
)
initial_llm_chain = LLMChain(llm=llm, prompt=prompt)
initial_response_name = "prev_response"
# The prompt here should take as an input variable the
# `document_variable_name` as well as `initial_response_name`
prompt_refine = PromptTemplate.from_template(
"Here's your first summary: {prev_response}. "
"Now add to it based on the following context: {context}"
)
refine_llm_chain = LLMChain(llm=llm, prompt=prompt_refine)
chain = RefineDocumentsChain(
initial_llm_chain=initial_llm_chain,
refine_llm_chain=refine_llm_chain,
document_prompt=document_prompt,
document_variable_name=document_variable_name,
initial_response_name=initial_response_name,
)
"""
initial_llm_chain: LLMChain
"""LLM chain to use on initial document."""
refine_llm_chain: LLMChain
"""LLM chain to use when refining."""
document_variable_name: str
"""The variable name in the initial_llm_chain to put the documents in.
If only one variable in the initial_llm_chain, this need not be provided."""
initial_response_name: str
"""The variable name to format the initial response in when refining."""
document_prompt: BasePromptTemplate = Field(
default_factory=_get_default_document_prompt
)
"""Prompt to use to format each document, gets passed to `format_document`."""
return_intermediate_steps: bool = False
"""Return the results of the refine steps in the output."""
...
def combine_docs(
self, docs: List[Document], callbacks: Callbacks = None, **kwargs: Any
) -> Tuple[str, dict]:
"""Combine by mapping first chain over all, then stuffing into final chain.
Args:
docs: List of documents to combine
callbacks: Callbacks to be passed through
**kwargs: additional parameters to be passed to LLM calls (like other
input variables besides the documents)
Returns:
The first element returned is the single string output. The second
element returned is a dictionary of other keys to return.
"""
inputs = self._construct_initial_inputs(docs, **kwargs)
res = self.initial_llm_chain.predict(callbacks=callbacks, **inputs)
refine_steps = [res]
for doc in docs[1:]:
base_inputs = self._construct_refine_inputs(doc, res)
inputs = {**base_inputs, **kwargs}
res = self.refine_llm_chain.predict(callbacks=callbacks, **inputs)
refine_steps.append(res)
return self._construct_result(refine_steps, res)
def _construct_result(self, refine_steps: List[str], res: str) -> Tuple[str, dict]:
if self.return_intermediate_steps:
extra_return_dict = {"intermediate_steps": refine_steps}
else:
extra_return_dict = {}
return res, extra_return_dict
def _construct_refine_inputs(self, doc: Document, res: str) -> Dict[str, Any]:
return {
self.document_variable_name: format_document(doc, self.document_prompt),
self.initial_response_name: res,
}
def _construct_initial_inputs(
self, docs: List[Document], **kwargs: Any
) -> Dict[str, Any]:
base_info = {"page_content": docs[0].page_content}
base_info.update(docs[0].metadata)
document_info = {k: base_info[k] for k in self.document_prompt.input_variables}
base_inputs: dict = {
self.document_variable_name: self.document_prompt.format(**document_info)
}
inputs = {**base_inputs, **kwargs}
return inputs
...
- The
combine_docs
method performs the following steps:- Generates a summary for the first document using
initial_llm_chain
. - For each subsequent document, it refines the summary using
refine_llm_chain
and the current document. - Uses
document_prompt
for pre-processing each document before summarization.-
The default template for
document_prompt
is as follows:PromptTemplate(input_variables=["page_content"], template="{page_content}")
-
- Returns the final refined summary.
- Generates a summary for the first document using
Summary
The load_summarize_chain
with chain_type="refine"
works as follows:
-
First, it summarizes the first document using
llm
andquestion_prompt
.-
The default
question_prompt
template is:Write a concise summary of the following: "{text}" CONCISE SUMMARY:
-
-
For each remaining document, it refines the summary using
refine_llm
andrefine_prompt
.-
If
refine_llm
is not specified,llm
is used. -
The default
refine_prompt
template is:Your job is to produce a final summary. We have provided an existing summary up to a certain point: {existing_answer} We have the opportunity to refine the existing summary (only if needed) with some more context below. ------------ {text} ------------ Given the new context, refine the original summary. If the context isn't useful, return the original summary.
-
-
The
document_prompt
can be specified to preprocess documents before summarization.