Reading LangChain's Summarization Code (1) - Stuff
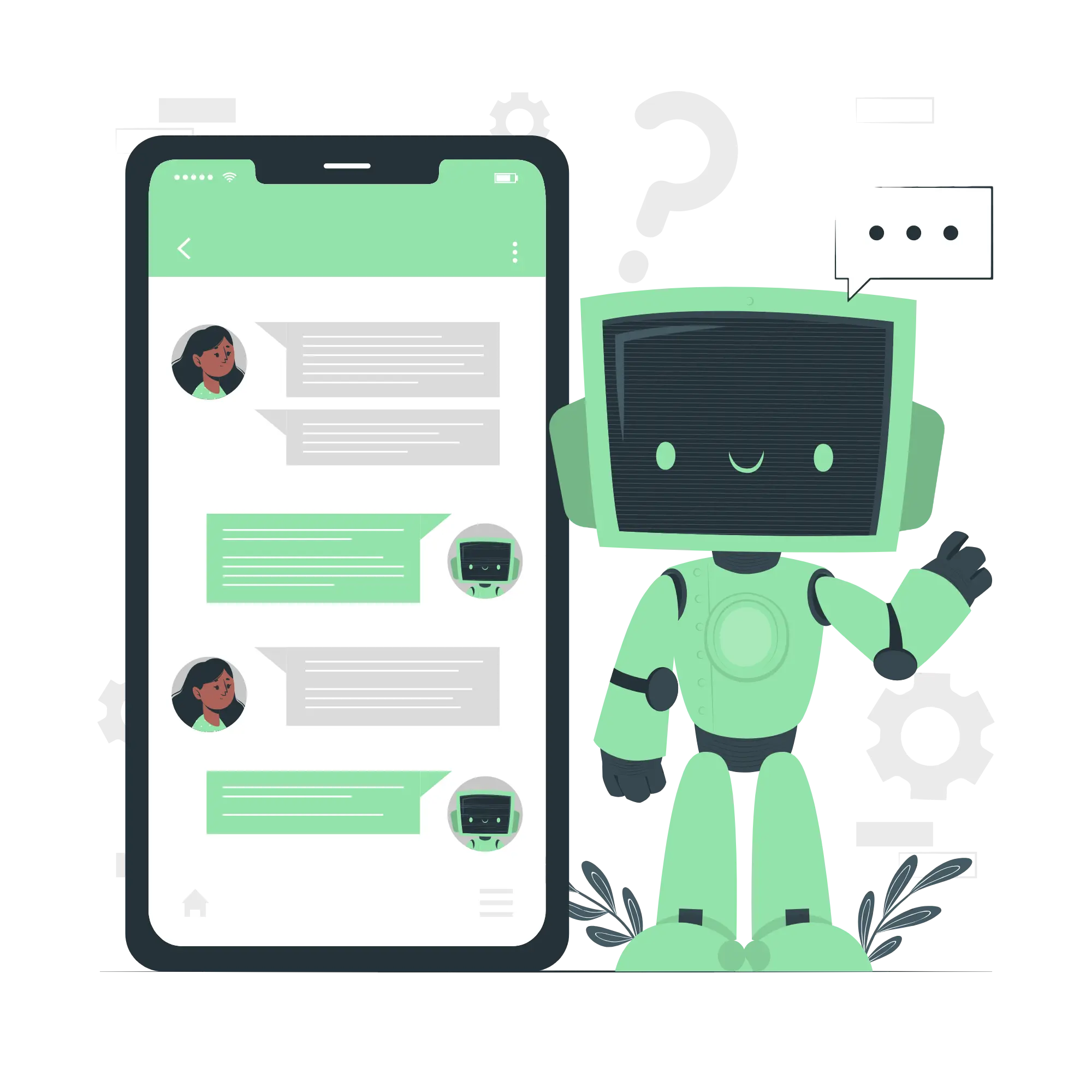
In this post, we’ll explore how the summarization chain in LangChain works, as outlined in the LangChain documentation on Summarization.
-
This article uses LangChain version
0.1.17
.$ pip list|grep langchain langchain 0.1.17 langchain-community 0.0.37 langchain-core 0.1.52 langchain-openai 0.1.6 langchain-text-splitters 0.0.1 langchainhub 0.1.15
1. Try Summarization
First, let’s take a look at the summarization code introduced in the Quickstart section of the documentation.
As we did in a series of previous posts, Exploring LangChain’s Quickstart, we’ll save the OpenAI API key in a .openai
file and load it into the OPENAI_API_KEY
environment variable.
import os
from langchain.chains.summarize import load_summarize_chain
from langchain_community.document_loaders import WebBaseLoader
from langchain_openai import ChatOpenAI
# Set the API key
with open('.openai') as f:
os.environ['OPENAI_API_KEY'] = f.read().strip()
# Load the document
loader = WebBaseLoader("https://lilianweng.github.io/posts/2023-06-23-agent/")
docs = loader.load()
# Set up the LLM
llm = ChatOpenAI(temperature=0, model_name="gpt-3.5-turbo-1106")
# Create the summarization chain
chain = load_summarize_chain(llm, chain_type="stuff")
# Execute the summarization
chain.run(docs)
Running this code produces the following summary:
'The article discusses the concept of LLM-powered autonomous agents, focusing on planning, memory, and tool use components. It includes case studies and proof-of-concept examples, as well as challenges and references to related research. The author also provides a citation for the article.'
- The
load_summarize_chain
function is used to create a chain that performs summarization.- The
chain_type
option is set tostuff
, butmap_reduce
andrefine
are also available options.
- The
Next, let’s dive deeper into how load_summarize_chain
works and explore each option.
2. Examine the load_summarize_chain
Code
The load_summarize_chain
function is defined as follows (link):
def load_summarize_chain(
llm: BaseLanguageModel,
chain_type: str = "stuff",
verbose: Optional[bool] = None,
**kwargs: Any,
) -> BaseCombineDocumentsChain:
"""Load summarizing chain.
Args:
llm: Language Model to use in the chain.
chain_type: Type of document combining chain to use. Should be one of "stuff",
"map_reduce", and "refine".
verbose: Whether chains should be run in verbose mode or not. Note that this
applies to all chains that make up the final chain.
Returns:
A chain to use for summarizing.
"""
loader_mapping: Mapping[str, LoadingCallable] = {
"stuff": _load_stuff_chain,
"map_reduce": _load_map_reduce_chain,
"refine": _load_refine_chain,
}
if chain_type not in loader_mapping:
raise ValueError(
f"Got unsupported chain type: {chain_type}. "
f"Should be one of {loader_mapping.keys()}"
)
return loader_mapping[chain_type](llm, verbose=verbose, **kwargs)
-
The function executed is determined by the
chain_type
parameter.chain_type Function stuff _load_stuff_chain
map_reduce _load_map_reduce_chain
refine _load_refine_chain
-
Each function is called with the parameters
llm, verbose=verbose, **kwargs
.
3. Option 1: Stuff
Let’s first examine the code for chain_type="stuff"
. The summarization chain is generated with the following code:
_load_stuff_chain(llm, verbose=verbose, **kwargs)
3.1. _load_stuff_chain
The _load_stuff_chain
function is defined as follows (link):
def _load_stuff_chain(
llm: BaseLanguageModel,
prompt: BasePromptTemplate = stuff_prompt.PROMPT,
document_variable_name: str = "text",
verbose: Optional[bool] = None,
**kwargs: Any,
) -> StuffDocumentsChain:
llm_chain = LLMChain(llm=llm, prompt=prompt, verbose=verbose) # type: ignore[arg-type]
# TODO: document prompt
return StuffDocumentsChain(
llm_chain=llm_chain,
document_variable_name=document_variable_name,
verbose=verbose, # type: ignore[arg-type]
**kwargs,
)
-
The
LLMChain
class is used to create a chain,llm_chain
, that generates responses using the specified LLM (llm
) and prompt template (prompt
).-
By default,
prompt
is set tostuff_prompt.PROMPT
, which is defined as follows (link):from langchain_core.prompts import PromptTemplate prompt_template = """Write a concise summary of the following: "{text}" CONCISE SUMMARY:""" PROMPT = PromptTemplate(template=prompt_template, input_variables=["text"])
-
-
The
StuffDocumentsChain
class is used to create a chain that performs summarization, passingllm_chain
to it.
3.2. StuffDocumentsChain
Next, let’s look at the StuffDocumentsChain
class code.
The StuffDocumentsChain
class is defined as follows (link):
class StuffDocumentsChain(BaseCombineDocumentsChain):
"""Chain that combines documents by stuffing into context.
This chain takes a list of documents and first combines them into a single string.
It does this by formatting each document into a string with the `document_prompt`
and then joining them together with `document_separator`. It then adds that new
string to the inputs with the variable name set by `document_variable_name`.
Those inputs are then passed to the `llm_chain`.
Example:
.. code-block:: python
from langchain.chains import StuffDocumentsChain, LLMChain
from langchain_core.prompts import PromptTemplate
from langchain_community.llms import OpenAI
# This controls how each document will be formatted. Specifically,
# it will be passed to `format_document` - see that function for more
# details.
document_prompt = PromptTemplate(
input_variables=["page_content"],
template="{page_content}"
)
document_variable_name = "context"
llm = OpenAI()
# The prompt here should take as an input variable the
# `document_variable_name`
prompt = PromptTemplate.from_template(
"Summarize this content: {context}"
)
llm_chain = LLMChain(llm=llm, prompt=prompt)
chain = StuffDocumentsChain(
llm_chain=llm_chain,
document_prompt=document_prompt,
document_variable_name=document_variable_name
)
"""
llm_chain: LLMChain
"""LLM chain which is called with the formatted document string,
along with any other inputs."""
document_prompt: BasePromptTemplate = Field(
default_factory=lambda: DEFAULT_DOCUMENT_PROMPT
)
"""Prompt to use to format each document, gets passed to `format_document`."""
document_variable_name: str
"""The variable name in the llm_chain to put the documents in.
If only one variable in the llm_chain, this need not be provided."""
document_separator: str = "\n\n"
"""The string with which to join the formatted documents"""
...
def _get_inputs(self, docs: List[Document], **kwargs: Any) -> dict:
"""Construct inputs from kwargs and docs.
Format and then join all the documents together into one input with name
`self.document_variable_name`. Also pluck any additional variables
from **kwargs.
Args:
docs: List of documents to format and then join into single input
**kwargs: additional inputs to chain, will pluck any other required
arguments from here.
Returns:
dictionary of inputs to LLMChain
"""
# Format each document according to the prompt
doc_strings = [format_document(doc, self.document_prompt) for doc in docs]
# Join the documents together to put them in the prompt.
inputs = {
k: v
for k, v in kwargs.items()
if k in self.llm_chain.prompt.input_variables
}
inputs[self.document_variable_name] = self.document_separator.join(doc_strings)
return inputs
...
def combine_docs(
self, docs: List[Document], callbacks: Callbacks = None, **kwargs: Any
) -> Tuple[str, dict]:
"""Stuff all documents into one prompt and pass to LLM.
Args:
docs: List of documents to join together into one variable
callbacks: Optional callbacks to pass along
**kwargs: additional parameters to use to get inputs to LLMChain.
Returns:
The first element returned is the single string output. The second
element returned is a dictionary of other keys to return.
"""
inputs = self._get_inputs(docs, **kwargs)
# Call predict on the LLM.
return self.llm_chain.predict(callbacks=callbacks, **inputs), {}
...
-
The docstring explains that the chain combines a list of documents into a single string using a prompt (
document_prompt
) and joins them with a separator (document_separator
). This combined string is then passed tollm_chain
for summarization. -
The summarization is done using the
combine_docs
method (see the Appendix for details). -
By default,
document_prompt
is set toDEFAULT_DOCUMENT_PROMPT
.document_prompt: BasePromptTemplate = Field( default_factory=lambda: DEFAULT_DOCUMENT_PROMPT )
-
DEFAULT_DOCUMENT_PROMPT
is defined as follows (link):PromptTemplate.from_template("{page_content}")
-
-
The
document_separator
is set to"\n\n"
by default.
3.3. Summary
The stuff
option for load_summarize_chain
works as follows:
-
The chain generated combines a list of documents into a single string and passes it to the LLM for summarization.
- This means it cannot summarize documents that exceed the length limit of the specified LLM.
-
The
prompt
option allows specifying a template for summarization.-
If not specified, the default template is used:
Write a concise summary of the following: "{text}" CONCISE SUMMARY:
-
-
The
document_prompt
option allows specifying a template for formatting each document.- If not specified, the default template is used, which outputs the document content as is.
-
The
document_separator
option allows specifying a separator for joining formatted documents.- If not specified,
"\n\n"
is used.
- If not specified,
Appendix
For those interested in more detailed information, here are some additional insights.
Chain’s invoke
Method
Let’s first look at the code for the invoke
method in the Chain
class. It is defined as follows (link):
class Chain(RunnableSerializable[Dict[str, Any], Dict[str, Any]], ABC):
"""Abstract base class for creating structured sequences of calls to components.
Chains should be used to encode a sequence of calls to components like
models, document retrievers, other chains, etc., and provide a simple interface
to this sequence.
The Chain interface makes it easy to create apps that are:
- Stateful: add Memory to any Chain to give it state,
- Observable: pass Callbacks to a Chain to execute additional functionality,
like logging, outside the main sequence of component calls,
- Composable: the Chain API is flexible enough that it is easy to combine
Chains with other components, including other Chains.
The main methods exposed by chains are:
- `__call__`: Chains are callable. The `__call__` method is the primary way to
execute a Chain. This takes inputs as a dictionary and returns a
dictionary output.
- `run`: A convenience method that takes inputs as args/kwargs and returns the
output as a string or object. This method can only be used for a subset of
chains and cannot return as rich of an output as `__call__`.
"""
...
def invoke(
self,
input: Dict[str, Any],
config: Optional[RunnableConfig] = None,
**kwargs: Any,
) -> Dict[str, Any]:
config = ensure_config(config)
callbacks = config.get("callbacks")
tags = config.get("tags")
metadata = config.get("metadata")
run_name = config.get("run_name") or self.get_name()
include_run_info = kwargs.get("include_run_info", False)
return_only_outputs = kwargs.get("return_only_outputs", False)
inputs = self.prep_inputs(input)
callback_manager = CallbackManager.configure(
callbacks,
self.callbacks,
self.verbose,
tags,
self.tags,
metadata,
self.metadata,
)
new_arg_supported = inspect.signature(self._call).parameters.get("run_manager")
run_manager = callback_manager.on_chain_start(
dumpd(self),
inputs,
name=run_name,
)
try:
self._validate_inputs(inputs)
outputs = (
self._call(inputs, run_manager=run_manager)
if new_arg_supported
else self._call(inputs)
)
final_outputs: Dict[str, Any] = self.prep_outputs(
inputs, outputs, return_only_outputs
)
except BaseException as e:
run_manager.on_chain_error(e)
raise e
run_manager.on_chain_end(outputs)
if include_run_info:
final_outputs[RUN_KEY] = RunInfo(run_id=run_manager.run_id)
return final_outputs
The invoke
method roughly follows these steps:
- It preprocesses the input data using the
prep_inputs
method. - It passes the preprocessed data to the
_call
method for execution. - It postprocesses the results using the
prep_outputs
method.
Base Class for Summarization Chains BaseCombineDocumentsChain
Chains that perform summarization, like StuffDocumentsChain
, inherit from the BaseCombineDocumentsChain
class. The _call
method in this class is defined as follows (link):
class BaseCombineDocumentsChain(Chain, ABC):
"""Base interface for chains combining documents.
Subclasses of this chain deal with combining documents in a variety of
ways. This base class exists to add some uniformity in the interface these types
of chains should expose. Namely, they expect an input key related to the documents
to use (default `input_documents`), and then also expose a method to calculate
the length of a prompt from documents (useful for outside callers to use to
determine whether it's safe to pass a list of documents into this chain or whether
that will be longer than the context length).
"""
...
def _call(
self,
inputs: Dict[str, List[Document]],
run_manager: Optional[CallbackManagerForChainRun] = None,
) -> Dict[str, str]:
"""Prepare inputs, call combine docs, prepare outputs."""
_run_manager = run_manager or CallbackManagerForChainRun.get_noop_manager()
docs = inputs[self.input_key]
# Other keys are assumed to be needed for LLM prediction
other_keys = {k: v for k, v in inputs.items() if k != self.input_key}
output, extra_return_dict = self.combine_docs(
docs, callbacks=_run_manager.get_child(), **other_keys
)
extra_return_dict[self.output_key] = output
return extra_return_dict
- The
_call
method processes the input document list using thecombine_docs
method.
Pydantic’s BaseModel
The Chain
class inherits from BaseModel
provided by pydantic.
Chain <- RunnableSerializable <- Serializable <- BaseModel
This means that parameters specified during instance creation (e.g., llm_chain
) are assigned to class variables with the same name. For more details, refer to the pydantic documentation.